ChexSystems in 2024 How Banks Are Refining Their Risk Assessment Strategies
ChexSystems in 2024 How Banks Are Refining Their Risk Assessment Strategies - AI-powered algorithms enhance ChexSystems scoring accuracy
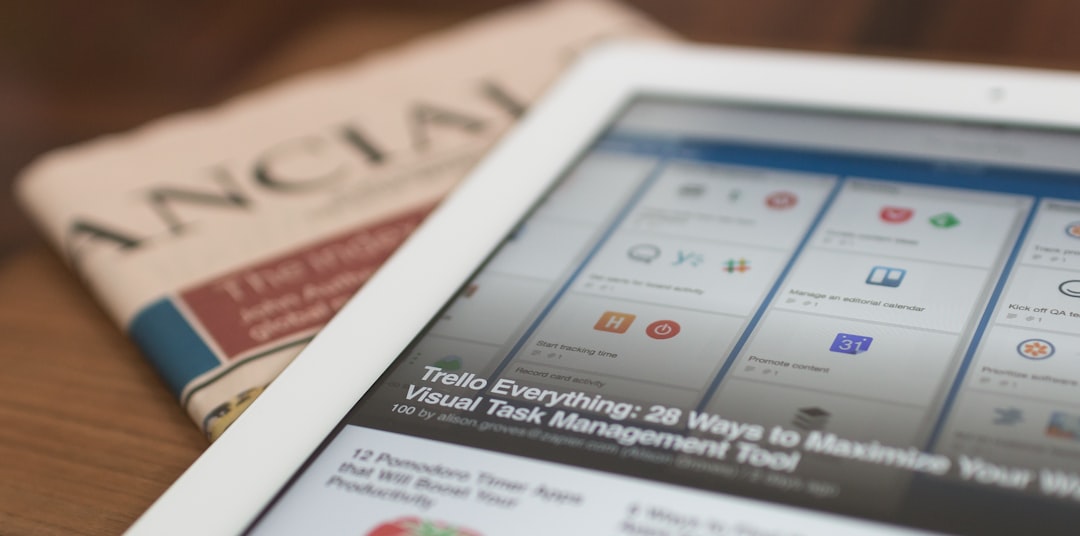
In 2024, ChexSystems' scoring system is undergoing a significant transformation with the integration of AI-powered algorithms. These algorithms are designed to refine the accuracy of the Consumer Score, a metric ranging from 100 to 899 that signifies a consumer's credit risk. By incorporating AI, the likelihood of inaccurate credit approvals or rejections is minimized, leading to more reliable assessments.
These AI models analyze a broader array of data points, providing a more nuanced understanding of individual creditworthiness. This ability to consider various factors allows for risk evaluations that go beyond traditional statistical methods, potentially uncovering subtle patterns or risks that might otherwise be missed. Beyond accuracy, the integration of AI also streamlines the assessment process, automating tasks and enabling quicker results. This efficiency, in turn, allows financial institutions to focus their resources on more complex cases and strategic decision-making.
The adoption of AI-driven credit scoring marks a fundamental change in how banks manage risk. While it promises improved accuracy and efficiency, it's important to remember that the reliance on complex algorithms necessitates careful consideration of potential biases and the need for transparency in how these scores are generated. The shift towards machine learning-based credit scoring could represent a positive development for both consumers and financial institutions if implemented and monitored thoughtfully.
ChexSystems' scoring system has seen a significant shift towards AI, leveraging algorithms to scrutinize a broader range of data. This includes not just the usual historical credit information but also transaction details and patterns of consumer behavior. This expanded view potentially offers a more comprehensive understanding of a consumer's risk profile, going beyond the limitations of traditional scoring methods.
The AI algorithms use advanced pattern recognition techniques to uncover subtle relationships in the data that may not be immediately obvious to human analysts. This capability can refine the precision of predicting future financial actions, leading to a reduction in inaccurate approvals or rejections. These algorithms also demonstrate adaptability to shifting trends in the financial landscape and consumer behaviors. As new data becomes available, the AI models can adjust the scoring system dynamically, maintaining its relevance over time.
A key feature of this AI approach is its ability to incorporate real-time information on spending and account activity, painting a more nuanced picture of a person's financial health compared to relying solely on past data. This approach also seems to be improving the identification of fraudulent behavior, potentially minimizing unnecessary flags for legitimate customers and streamlining the decision process for banks.
While the improved scoring benefits customers by providing more relevant financial products and services, this technological shift also raises some concerns. Maintaining data privacy and security is paramount, given the sensitive nature of the information being analyzed. There's also the question of how the evolving legal and regulatory landscape will shape the use of AI in risk assessment, especially given a need to balance innovation with ensuring fairness and transparency.
Finally, some users may be uneasy about the "black box" nature of AI-based decisions, prompting discussions on ensuring responsibility and explainability in these financial systems. Despite this uncertainty, the move towards AI-driven credit assessments appears to be a major development in banking risk management, though its broader impact and consequences are still being explored and debated.
ChexSystems in 2024 How Banks Are Refining Their Risk Assessment Strategies - Banks integrate real-time data sources for comprehensive risk profiles
In today's banking landscape, the need for comprehensive risk assessment has never been more pronounced. Banks are increasingly recognizing the limitations of relying solely on historical data and are embracing the integration of real-time data sources. This shift allows them to construct more complete and dynamic profiles of their customers, incorporating current financial behaviors and trends into their risk models. This move towards real-time data involves tapping into various sources like transaction details, spending patterns, and other relevant information. The ability to incorporate such diverse information is further fueled by advancements in technology like AI, which enables banks to sift through vast datasets and make rapid adjustments to risk management strategies. This means banks can potentially react more quickly to shifts in risk profiles and emergent threats.
While these advancements offer the enticing prospect of improved risk assessments and operational efficiency, they also bring a set of considerations. One primary concern involves the sensitive nature of the data being collected and the responsibility of banks to safeguard it from unauthorized access or misuse. There are also questions around the potential for biases inherent in AI-driven algorithms. If not carefully designed and monitored, such algorithms could lead to unfair or inaccurate assessments, harming individuals and communities. And, it's also crucial to consider how transparency and explainability are woven into this emerging risk management paradigm. Customers deserve clear answers about how their data is used in risk assessment, even when advanced technologies play a central role. Banks must navigate these complex issues carefully as they further develop and deploy these powerful new tools for evaluating risk.
Banks are increasingly relying on a wider range of real-time data sources to build more complete and dynamic pictures of their customers' risk profiles. This means incorporating continuous streams of data—think transaction histories, spending patterns, and other behavioral clues—into their risk assessment processes. This approach offers the potential to make much more accurate predictions about a customer's creditworthiness compared to older methods that solely relied on historical data. This real-time view provides a level of agility that was simply not possible with the older, more static models.
Beyond just financial history, these new algorithms are starting to incorporate things like spending behaviors into the risk assessment. This more multi-faceted analysis can help uncover potential risks or opportunities that might be missed by traditional methods that primarily looked at traditional credit reports. The result is a more nuanced understanding of who a borrower really is, and that can lead to better decisions for both banks and customers.
The move towards real-time data brings with it significant benefits when it comes to detecting fraudulent activity. Banks can now monitor transactions as they happen, allowing for the rapid identification of any anomalies that might suggest a fraudulent attempt. This real-time detection cuts down on the time it takes to respond to suspicious activity, which is a definite improvement over how things used to be handled.
However, this increase in the sheer volume of data raises some important questions. Can our current infrastructure handle these massive datasets without slowing down our systems or affecting the customer experience? How can we make sure that our systems can efficiently process the massive influx of information without sacrificing performance? This shift towards more data will need to be accompanied by an expansion and adaptation of the underlying infrastructure if the potential benefits are to be fully realized.
This new, more complete data profile of borrowers also has implications for how banks interact with their customers. With access to a real-time understanding of a customer's finances, banks can offer financial products and services that are tailored to those specific circumstances. This ability to personalize the experience can foster a greater level of engagement with customers, as they'll feel the bank has a more intimate and relevant understanding of their individual financial needs.
Yet, as we rely more and more on these AI-driven and data-driven systems, it's important to acknowledge the need for increased transparency. Banks are now faced with the task of communicating the processes of these sophisticated algorithms and risk models to their customers in a way that is accessible and understandable. The goal is to address concerns and build trust, which becomes all the more important as the decision-making processes become more complex and automated.
Furthermore, the shift towards real-time data raises compliance concerns within the ever-evolving legal and regulatory environment. As banks collect and use this vast amounts of personal data, they need to continually innovate their compliance procedures and strategies to keep up with the increasingly stringent guidelines that govern this field. Meeting these guidelines without impeding innovation is a tough challenge.
One of the most obvious costs associated with this adoption of real-time data is the investment needed in upgrading technology and building the systems to support these advanced processes. The upfront costs can be significant, which can pose a considerable barrier for smaller banks that might not have the resources of larger institutions. But, there's a strong possibility that the decreased number of loan defaults could lead to reduced risk management expenses in the long run.
As we integrate more external data streams, there's a risk of introducing unforeseen correlations or connections between different risk factors. The interwoven nature of the data itself can create new and unexpected sources of risk that weren't apparent in more isolated approaches. Understanding how to analyze these connections and build a strategy to minimize potential vulnerabilities will be a critical next step.
The shift towards using more advanced real-time data analytics also amplifies the already existing gap in the skillsets required in the banking industry. The demand for data scientists and analysts capable of handling large data sets continues to grow, highlighting a need to address training and education to ensure banks can staff up appropriately for this future of risk management.
ChexSystems in 2024 How Banks Are Refining Their Risk Assessment Strategies - Blockchain technology improves security and transparency in ChexSystems
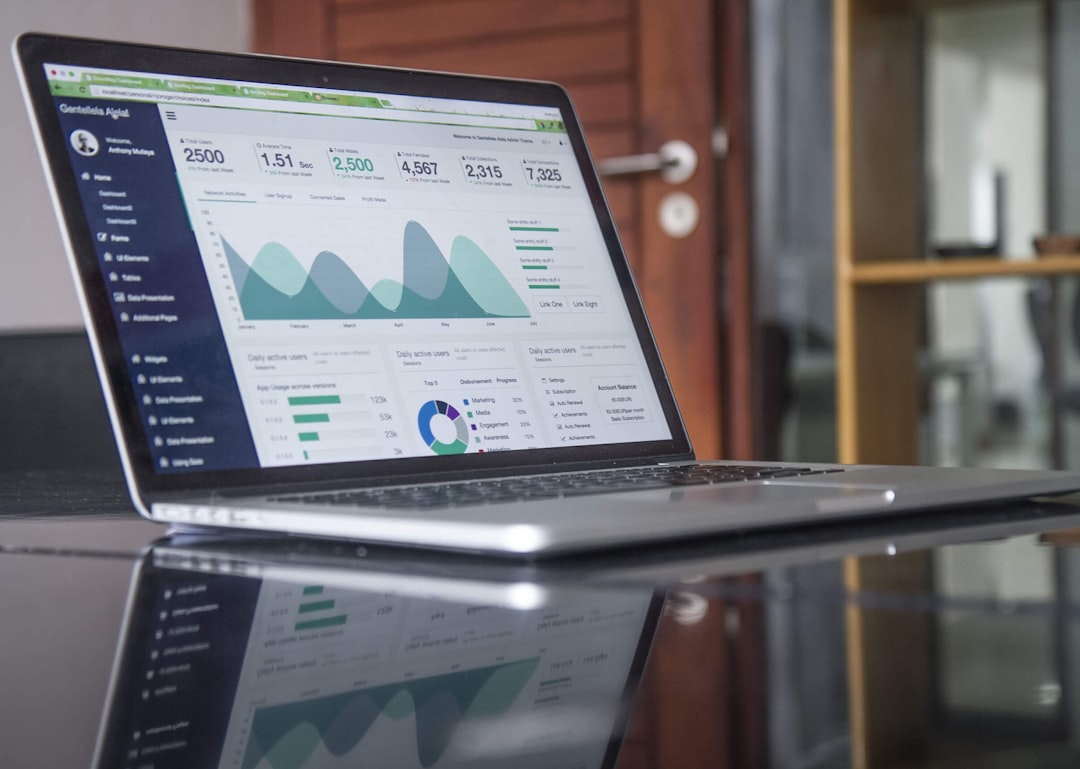
In the evolving banking landscape of 2024, ChexSystems is exploring the potential of blockchain technology to bolster security and transparency. The inherent nature of blockchain, with its distributed ledger, could lead to more secure and tamper-proof records of consumer banking history and transactions. This enhanced transparency could, in theory, foster trust between consumers and financial institutions. Additionally, the immutability of blockchain data could help reduce the risk of fraud and data breaches.
However, the implementation of blockchain in such a complex system as ChexSystems comes with its own set of challenges. Security vulnerabilities related to blockchain technology need to be carefully addressed. Moreover, seamlessly integrating this novel technology into existing systems could prove complex and potentially disruptive. As banks continue to refine their risk assessment strategies, balancing the need for increased transparency with the importance of secure data handling is an ongoing and critical concern.
Blockchain technology is increasingly being explored as a way to improve the security and transparency of systems like ChexSystems. Its decentralized nature creates a shared, immutable record of all transactions, making it incredibly difficult for anyone to tamper with data. This could lead to a significant reduction in fraudulent activity since altering records would be nearly impossible to do without detection.
Another potential benefit is increased transparency for consumers. Imagine if consumers could see, in real time, who has accessed their ChexSystems data and why. This type of access could foster trust by giving consumers more control and insight into how their information is being used.
Furthermore, every transaction on a blockchain is time-stamped and linked to previous transactions, forming a detailed and easily verifiable audit trail. This feature could simplify regulatory compliance for banks relying on ChexSystems data, since they could readily demonstrate that their use of this data is compliant with relevant regulations.
The process of validating transactions on a blockchain often involves a consensus mechanism, requiring multiple parties to agree on the validity of a transaction before it's recorded. This distributed validation can significantly minimize errors and ensure the accuracy of the data—a crucial factor for banks using the data for credit scoring.
Interestingly, blockchain technology also allows for the implementation of smart contracts, which are essentially automated agreements coded into the blockchain. In theory, this could automate a lot of the risk assessment processes currently handled by ChexSystems, leading to faster loan approvals or denials based on pre-set criteria.
However, there are potential roadblocks to widespread adoption of blockchain within ChexSystems. While it offers the potential for improved security, there are still challenges to overcome. Establishing standards and protocols across the banking industry for blockchain use in risk assessment is likely to be a significant hurdle. Without a clear and broadly accepted approach, it's hard to see how it can be fully integrated into systems like ChexSystems.
Furthermore, although blockchain offers encryption features to protect consumer data, the successful implementation of these safeguards will need to be carefully monitored and tested before becoming widely used. Ultimately, achieving a fully functional blockchain-based ChexSystems depends on the level of collaboration between banks and technology providers. While the technology promises a lot, its practical implementation within a complex financial system like ChexSystems will require time and careful consideration.
ChexSystems in 2024 How Banks Are Refining Their Risk Assessment Strategies - Regulatory changes prompt banks to adjust ChexSystems usage policies
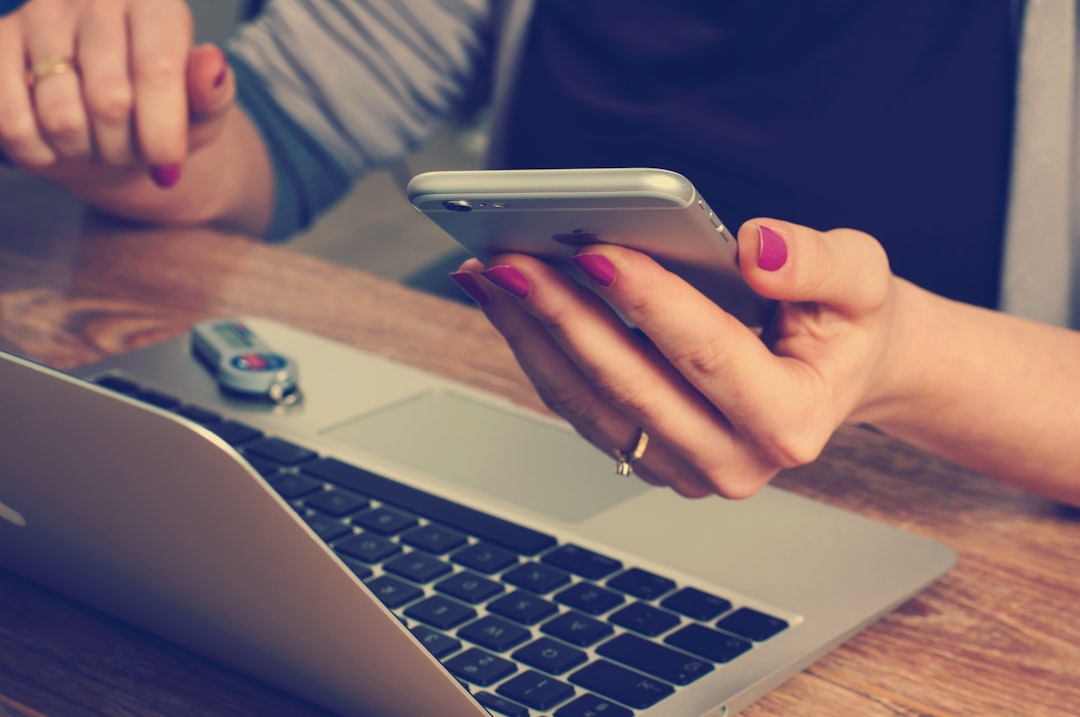
Recent regulatory shifts, particularly those focused on fair lending and consumer protection, are prompting banks to reevaluate how they use ChexSystems. As new regulations are expected to finalize in 2024, banks are realizing that ChexSystems' historical approach might have been too harsh on consumers with minor past financial issues. This increased scrutiny has highlighted the need for banks to rethink their risk assessment methods. The goal is to move away from a system that relies too heavily on past events and toward a more balanced approach that takes individual circumstances into account. Essentially, the aim is to build a fairer banking environment while ensuring that banks meet compliance standards and maintain consumer trust as the regulatory landscape continues to evolve. This adjustment reflects the ongoing tension between managing risk effectively and treating consumers equitably.
Changes in regulations are driving banks to rethink how they use ChexSystems. They're being pushed to adopt more adaptable approaches to evaluating customer risk, potentially lessening the impact of past negative events on account applications. This move seems to be driven by a growing understanding that rigid reliance on historical data can be unfair and potentially discriminatory.
Banks are under increasing pressure to be more transparent about how they utilize ChexSystems data. Many see transparency as a way to build trust with customers, which could give them a competitive edge. This emphasis on openness is a direct result of tighter regulations designed to protect consumer rights.
New regulations specifically focused on data privacy are making banks carefully consider how they gather, retain, and use customer data from ChexSystems. This push is geared towards ensuring bank practices are in sync with evolving consumer rights, which has introduced another layer of complexity to existing risk management practices.
The risk of using ChexSystems data improperly is now much higher for banks, leading them to put more emphasis on internal control processes and better training for employees. This shift in priorities is largely a response to increased penalties and legal risks that can arise from misusing sensitive data. It raises the question: how do we balance managing risk with the rights and needs of people seeking financial services?
Banks are increasingly supplementing ChexSystems data with real-time data sources. This trend has been fostered by regulators who want banks to take a more comprehensive view of a consumer's financial behavior rather than simply relying on past negative incidents. It will be interesting to see how this influences the decision-making processes in the future.
The push for regulatory compliance has also compelled banks to invest more heavily in tech upgrades to improve their ChexSystems processes. This redirection of capital toward innovation in risk evaluation is a clear signal of how these regulatory changes are prompting institutions to change how they operate. However, it is important to consider whether the investment in advanced technology can sufficiently address the underlying issues regarding the fairness and ethics of credit decisions.
Banks are being forced to meticulously scrutinize their algorithms for potential biases to ensure they're compliant with new regulations. This involves making sure their assessments based on ChexSystems data don't disadvantage specific population groups. It remains unclear how successful banks will be in eliminating these biases with greater scrutiny alone.
The latest updates in legislation have prompted a discussion about the ethical implications of using ChexSystems in risk assessment. This debate is leading banks to consider how they can better integrate their services with the needs of communities, potentially contributing to a broader conversation about fairness in the financial industry. This is a promising development that could result in real change or be simply a temporary reaction to external pressures.
Managing compliance with the new regulations regarding ChexSystems is a top concern for banking risk teams. This has led to the development of dedicated groups within these organizations that focus exclusively on adhering to these ever-evolving guidelines. The sheer scale and complexity of managing this aspect of risk evaluation represents a notable shift in the way banking institutions approach customer relationships.
Interestingly, the regulatory drive towards fairness in lending is also causing banks to build stronger partnerships with consumer advocacy groups. The goal of these partnerships is to refine ChexSystems policies to produce a more equitable risk assessment structure. It's critical that these initiatives focus on promoting both fairness and sustainability in the broader banking environment.
ChexSystems in 2024 How Banks Are Refining Their Risk Assessment Strategies - Alternative credit data expands risk assessment beyond traditional metrics
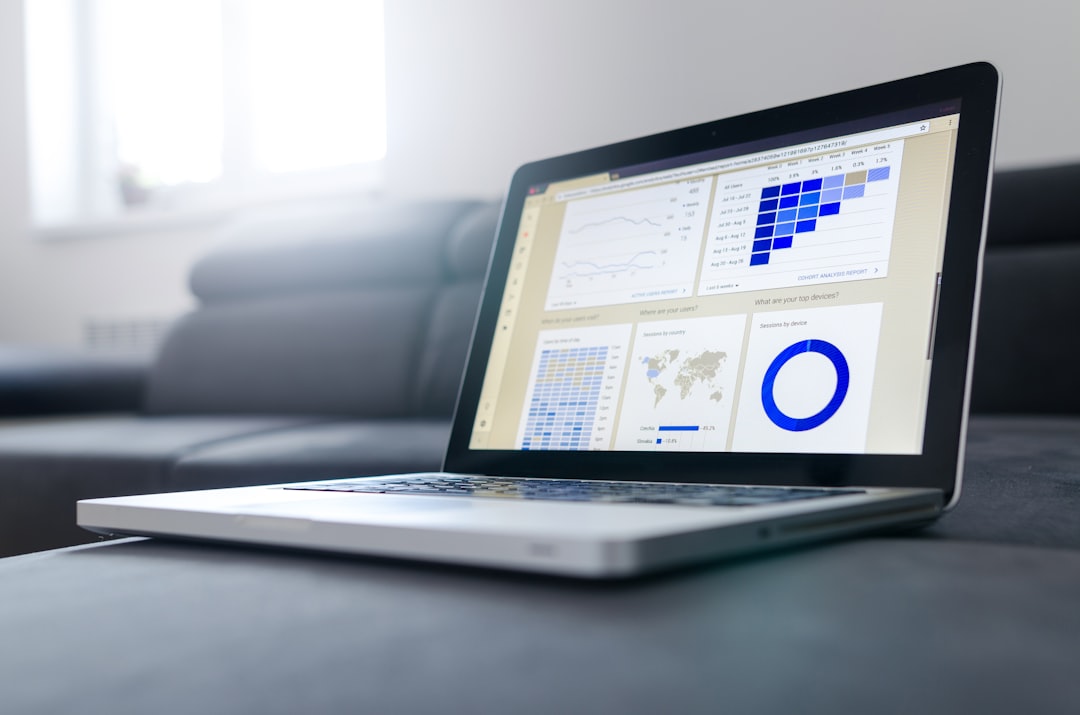
In 2024, banks are increasingly looking beyond traditional credit scores to assess risk, incorporating what's called "alternative credit data". This involves using a wider range of information, like social media activity, online behavior, and even customer service interactions, to get a better picture of a person's financial reliability. This shift is particularly helpful in areas where traditional credit reporting is limited or unavailable, potentially opening up access to credit for those who've historically been underserved by the financial system. But, integrating this new kind of data comes with its own hurdles. Banks need to overhaul how they operate, updating their systems and procedures to handle this different type of information. They also need to grapple with the complexities of ensuring fairness and protecting consumer privacy, especially as AI-driven algorithms become more involved in the assessment process. Ultimately, this broader approach to risk assessment creates a more complex landscape for credit scoring, making it crucial for banks to be cautious and thoughtful in how they implement these new methods. While there's potential for significant improvements in financial access, banks must carefully navigate these changes to make sure they're both beneficial and ethical.
Alternative credit data is gaining recognition as a powerful tool to go beyond the usual credit scores when figuring out how risky a borrower is. It's about using things like rent payments or utility bills, which offer a broader look at someone's money habits.
One interesting aspect is the possibility of helping people who haven't had much access to traditional credit. By considering things like mobile payment patterns or social media activity, banks might be able to give more people a chance to get loans. Some research suggests that combining traditional and alternative credit data can significantly boost the accuracy of predicting loan defaults, making risk management potentially much better.
These new credit scoring systems can adapt to changes in people's financial behavior, unlike the old methods that mainly relied on historical data. This is useful for banks that want to be quick on their feet when risk changes. Moreover, the use of alternative data has shown promise in improving fraud detection. For example, spotting out-of-the-ordinary spending patterns can flag suspicious behavior better than traditional methods.
Many of these new methods involve machine learning, allowing banks to spot complex patterns in huge amounts of data, which can be tough for people to do on their own. This helps in evaluating risks more accurately and quickly.
Of course, using alternative data also brings up ethical questions. Banks need to be careful about privacy and get permission from consumers before using their data. They need to understand the laws around how this data can be used.
Another benefit is that it can reduce the number of times someone is wrongly denied credit. By looking at the bigger picture of a person's finances, it's less likely that someone gets rejected simply because of a limited credit history.
Insights from alternative data can guide banks in ways that go beyond credit decisions. By seeing how someone saves or spends over time, banks can adjust their lending strategies for the broader customer base.
As the use of alternative data increases, banks are likely to invest more in technologies that handle all the new data they need to process. This will be essential to stay competitive in the future of banking, which is increasingly focused on data analysis. It will be interesting to see how these technological trends affect the overall financial landscape.
More Posts from :